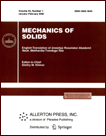 | | Mechanics of Solids A Journal of Russian Academy of Sciences | | Founded
in January 1966
Issued 6 times a year
Print ISSN 0025-6544 Online ISSN 1934-7936 |
Archive of Issues
Total articles in the database: | | 13025 |
In Russian (Èçâ. ÐÀÍ. ÌÒÒ): | | 8110
|
In English (Mech. Solids): | | 4915 |
|
<< Previous article | Volume 59, Issue 3 / 2024 | Next article >> |
Teng Wenxiang, Qian Cheng, Yan Leilei, Shen Gang, Liu Pengyu, He Jipeng, and Wang Cheng, "Research on Application of Backpropagation Neural Network in Damage Detection of the Refined Plate Model," Mech. Solids. 59 (3), 1672-1688 (2024) |
Year |
2024 |
Volume |
59 |
Number |
3 |
Pages |
1672-1688 |
DOI |
10.1134/S0025654424603392 |
Title |
Research on Application of Backpropagation Neural Network in Damage Detection of the Refined Plate Model |
Author(s) |
Teng Wenxiang (Anhui University of Science and Technology, Huainan, 232001 China; State Key Laboratory of Mining Response and Disaster Prevention and Control in Deep Coal Mines, Anhui University of Science and Technology, Huainan, 232001 China; Mining Intelligent Technology and Equipment Provinces and Ministries jointly build a Collaborative Innovation Center, Anhui University of Science and Technology, Huainan, 232001 China, wxtengcumt@163.com)
Qian Cheng (Anhui University of Science and Technology, Huainan, 232001 China; State Key Laboratory of Mining Response and Disaster Prevention and Control in Deep Coal Mines, Anhui University of Science and Technology, Huainan, 232001 China, 18326691883@163.com)
Yan Leilei (Anhui University of Science and Technology, Huainan, 232001 China; State Key Laboratory of Mining Response and Disaster Prevention and Control in Deep Coal Mines, Anhui University of Science and Technology, Huainan, 232001 China; Mining Intelligent Technology and Equipment Provinces and Ministries jointly build a Collaborative Innovation Center, Anhui University of Science and Technology, Huainan, 232001 China)
Shen Gang (Anhui University of Science and Technology, Huainan, 232001 China; State Key Laboratory of Mining Response and Disaster Prevention and Control in Deep Coal Mines, Anhui University of Science and Technology, Huainan, 232001 China; Mining Intelligent Technology and Equipment Provinces and Ministries jointly build a Collaborative Innovation Center, Anhui University of Science and Technology, Huainan, 232001 China)
Liu Pengyu (Anhui University of Science and Technology, Huainan, 232001 China; State Key Laboratory of Mining Response and Disaster Prevention and Control in Deep Coal Mines, Anhui University of Science and Technology, Huainan, 232001 China)
He Jipeng (Anhui University of Science and Technology, Huainan, 232001 China; State Key Laboratory of Mining Response and Disaster Prevention and Control in Deep Coal Mines, Anhui University of Science and Technology, Huainan, 232001 China)
Wang Cheng (Anhui University of Science and Technology, Huainan, 232001 China; State Key Laboratory of Mining Response and Disaster Prevention and Control in Deep Coal Mines, Anhui University of Science and Technology, Huainan, 232001 China) |
Abstract |
Artificial intelligence has been widely used in engineering. In this paper, we propose to
combine the backpropagation neural network (BPNN) with the refined plate model based on Carrera
Unified Formula (CUF) to advance the development of damage detection. The prediction model is
built by utilizing the error back propagation function of the neural network. In addition, MATLAB
uses Taylor’s interpolation algorithm and lower degrees of freedom yet achieves the same accuracy as
ANSYS, and the improved plate model accurately reproduces the mechanical properties of the metal
plate. A database is then built based on the mechanical model to detect the location of damaged elements and node displacements. The nodal displacements were used as inputs while the locations of
damaged elements were used as training outputs for the neural network. The effectiveness of the proposed method was verified through various damage scenarios. The results show that the method can
accurately predict individual damage locations based on node displacements alone. The neural network combined with the plate model achieved a detection accuracy of 91% with a regression coefficient of 0.95. |
Keywords |
damage detection, backpropagation neutral network (BPNN), carrera unified formulation (CUF), higher-order finite element |
Received |
17 April 2024 | Revised |
08 July 2024 | Accepted |
09 July 2024 |
Link to Fulltext |
|
<< Previous article | Volume 59, Issue 3 / 2024 | Next article >> |
|
If you find a misprint on a webpage, please help us correct it promptly - just highlight and press Ctrl+Enter
|
|